Organizations are increasingly relying on machine learning as a powerful tool to protect their sensitive information. Machine Learning (ML) takes center stage in this digital defense, actively preventing the accidental sharing of confidential data through improved email security and heightened user awareness.
Machine learning algorithms step in with remarkable accuracy, analyzing email content to identify sensitive information and prevent unauthorized sharing, even in scenarios where traditional rule-based systems may fall short. It's like having a vigilant guardian, ensuring the security of your data in the dynamic landscape of digital communication.
The problem lies inside: data breaches due to misdirected emails
While machine learning is reshaping our defense mechanisms, the challenge it addresses is anything but new. Data breaches resulting from misdirected emails have plagued organizations for years. This common and potentially damaging security incident involves sending sensitive information to the wrong person. Whether it's confidential business data, personal information, or financial details, the consequences of such missteps can be severe, ranging from reputational damage to legal ramifications.
Enter machine learning, a transformative technological evolution that is redefining the landscape of Data Loss Prevention (DLP).
Machine Learning's role in Accidental Data Loss Prevention
Data loss prevention (DLP) refers to the set of policies and procedures that organizations implement to prevent the unauthorized disclosure of sensitive data. Machine learning plays a crucial role in enhancing DLP mechanisms. By analyzing the context of emails – considering factors such as sender, recipient, and content – machine learning algorithms offer a more nuanced approach to identifying sensitive information. This contextual understanding empowers machine learning to detect potential data breaches even when sensitive data is not explicitly mentioned in the email content, marking a significant leap forward in the ongoing battle against outbound email-related security incidents.
Furthermore, the capability of machine learning to deliver interpretability (focusing on understanding the inner workings of the models) and explainability (focused on explaining the decisions made) significantly elevates the effectiveness of Accidental Data Loss Prevention mechanisms. This not only enhances the accuracy of DLP but also plays a crucial role in increasing organizational awareness. The ability to explain flagged emails and pinpoint specific sensitive information at risk contributes to a more informed and nuanced approach to prevent unauthorized disclosure. This not only signifies progress in countering outbound email-related security incidents but also fosters deeper organizational awareness by providing insights into potential threats and the underlying rationale behind DLP decisions.
The role of Machine Learning in email security
Machine learning algorithms play a pivotal role in fortifying email security by analyzing the context of messages and recognizing the sensitivity of their content. Here's how machine learning helps prevent data breaches caused by misdirected emails:
1. Contextual Analysis:
Machine learning algorithms excel at understanding the nuances of language and context. By analyzing the content of emails, these algorithms can identify patterns indicative of sensitive information. For instance, recognizing the mention of proprietary terms, financial figures, or personal identifiers allows the system to flag an email as potentially sensitive.
2. Automatic Protection:
Once an email is identified as containing sensitive information, machine learning systems can automatically trigger protective measures. This might involve encrypting the email or restricting access to only authorized recipients. Automation not only ensures swift response but also reduces the reliance on manual intervention, minimizing the window of vulnerability.
3. Reduced False Positives:
Traditional content policies often lead to a barrage of false positives, causing frustration among users who find their messages needlessly flagged as sensitive. Machine learning, on the other hand, is more accurate, which reduces the occurrence of false positives, ensuring that only sensitive content is flagged and secured.
Conclusion: harnessing Machine Learning for safer emails
As the digital threat landscape evolves, our defenses must evolve in tandem. Machine learning offers a dynamic and intelligent solution to the persistent challenge of preventing data breaches through misdirected emails. By analyzing the context, automatically triggering protective measures, and reducing false positives, machine learning transforms email security into a proactive and user-friendly safeguard.
In the ever-expanding digital ecosystem, integrating machine learning into email security practices is not just a technological enhancement but a strategic imperative for organizations committed to preserving the confidentiality and integrity of their sensitive information.
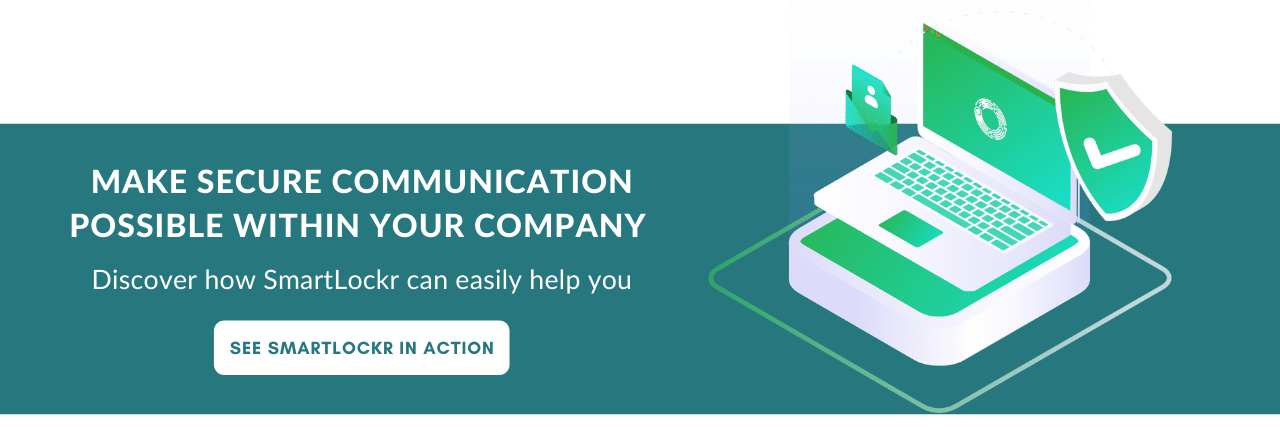